Parents, taxpayers, and policymakers everywhere are justly concerned about the quality of their local public school districts. In states slammed hardest by the financial crisis, like New York, it’s more important than ever to know which districts perform well with the resources they have and which don’t deliver enough bang for the taxpayers’ buck.
But assessing the efficiency of school districts isn’t as simple as comparing average test scores. Test scores measure student proficiency, true; but that proficiency is the product of many factors, not all of them under a district’s control. Some districts serve student populations with characteristics statistically associated with lower educational achievement: low family income, certain racial or ethnic backgrounds, lack of language proficiency. Some districts spend less per pupil than others do. Local environmental factors, such as the quantity of unemployment and single parenthood in an area, can also mean lower outcomes. To find the districts with the highest test scores, you usually need look no farther than those with the whitest, wealthiest students—but to determine which do the best with what they have, you must look deeper.
What we really want to know in measuring efficiency is how well a school district performs relative to what we would expect, given the characteristics of its students and the resources at its disposal. To calculate that, I have used a standard regression technique to create a model estimating the relationship between a district’s observed characteristics in the 2006–07 school year—such as size, average expenditure per pupil, racial and ethnic composition, income and demographic characteristics of the surrounding population, and so on—and the district’s average third- through eighth-grade test scores. Then I use these estimates to predict the average test score that each district might be expected to have, given its characteristics. The difference between this predicted average test score and the district’s actual average test score can be thought of as the influence of the school district itself. When the difference is positive—the actual score being higher than the prediction—the school is performing above expectations; when the difference is negative, it’s performing worse.
Because of space considerations, the accompanying tables combine New York State’s nine traditional regions (excluding the tenth, New York City, for complex methodological reasons) into five and list the most and least efficient districts in each. Westchester joins Long Island, to which it is more similar demographically than to the rest of the mid-Hudson area. The procedure is not exact enough to compare one school district with another precisely; we cannot point to two districts and calculate, say, that one is the region’s fifth most productive and that the other is the sixth. We can, however, show (in alphabetical order) the districts that place in the top and bottom 10 percent of each region.
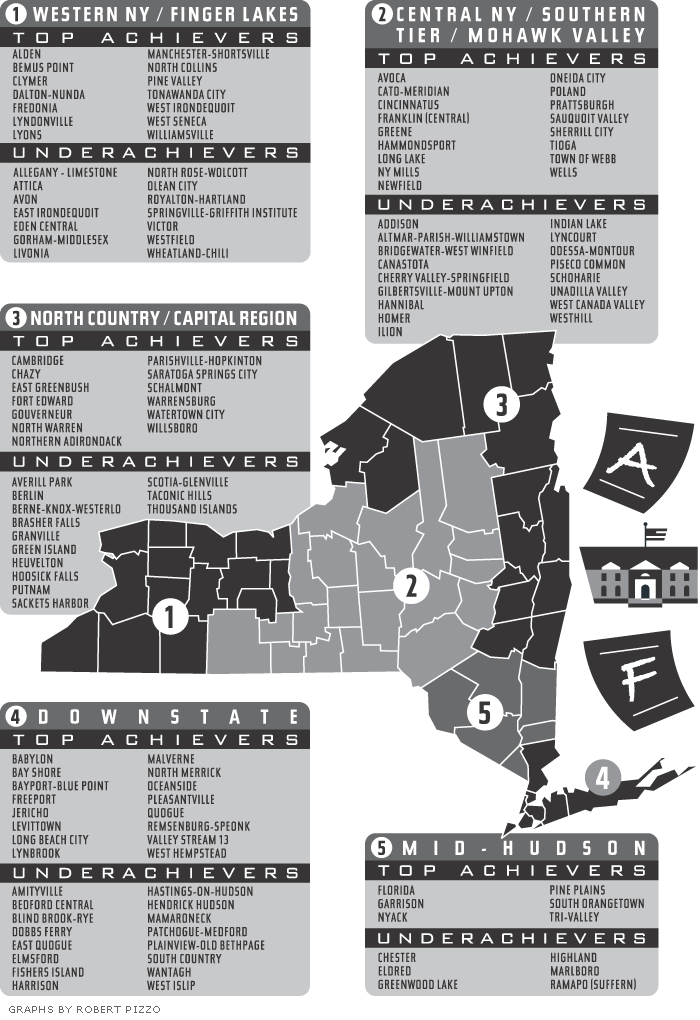